Stanford Alpaca: An Outstanding Contribution to the Medical Imaging World
The world of medical imaging has experienced significant evolution thanks to the advent of advanced technologies. A significant contribution in this regard has come in the shape of an open-source project aptly named 'Stanford Alpaca', hosted on the popular software development platform - GitHub. This project is the brainchild of Tatsu-lab, aiming to simplify radiological images' deep-learning tasks.
Project Overview:
'Stanford Alpaca' is an awe-inspiring project that aims at competent deep learning and rich visualization of radiological images. Its primary goal is to bridge the gap between the digital imaging world and radiologists by providing a platform where they can manage, visualize, and process a vast amount of radiological images through deep learning. The project serves to automate the process of analyzing these images, thereby saving time for the medical practitioners and increasing efficiency. Its potential users constitute researchers, radiologists, and healthcare organizations seeking to leverage deep learning for radiological image analysis.
Project Features:
'Stanford Alpaca' showcases numerous impressive features. Among those, its ability to facilitate an automated deep learning process for the analysis of radiological images holds the limelight. It also incorporates automated tracing, which proves to be a life-saver in tracing and marking images, thereby easing the process of diagnosis. Moreover, the project allows large-scale data handling, enabling users to effectively manage a high volume of images. This feature significantly reduces the burden of manual inspection and accelerates the diagnosing process.
Technology Stack:
Underpinning 'Stanford Alpaca' is a powerful technology stack that includes Python and OpenCV. Python, being a popular language for developing AI models, aids in building a robust and intelligent system capable of understanding and processing data more efficiently. OpenCV, with its wide-ranging functions dedicated to real-time image processing, contributes to the success of Stanford Alpaca by enhancing its ability to manage and process images.
Project Structure and Architecture:
The overall architecture of Stanford Alpaca revolves around its deep learning model and the management of radiological images. It operates through various components, including the image pre-processor, deep learning model, and visualization system. These components interact seamlessly, ensuring the smooth operation of the project, from ingesting the images to processing and analyzing them for comprehensive visualizations.
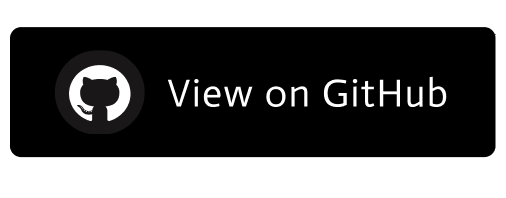